Report the ad
How to Interpret Regression Analysis Results: P-values & Coefficients? - Chennai
Tuesday, 11 April, 2017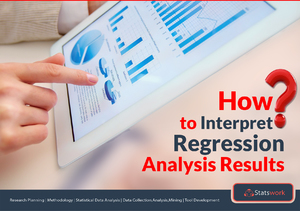
Item details
City:
Chennai, Tamil Nadu
Offer type:
Offer
Item description
Statistical Regression analysis provides an equation that explains the nature and relationship between the predictor variables and response variables. For a linear regression analysis, following are some of the ways in which inferences can be drawn based on the output of p-values and coefficients.
While interpreting the p-values in linear regression analysis in statistics, the p-value of each term decides the coefficient which if zero becomes a null hypothesis. A low p-value of less than .05 allows you to reject the null hypothesis. This could mean that if a predictor has a low p-value, it could be an effective addition to the model as the changes in the value of the predictor are directly proportional to the changes in the response variable.
On the contrary, a p-value that is larger does not affect the model as in that case, the changes in the value of the predictor and the changes in the response variable are not directly linked.
If you are to take an output specimen like given below, it is seen how the predictor variables of Mass and Energy are important because both their p-values are 0.000. Nevertheless, the p-value for Velocity is greater than the maximum common alpha level of 0.05 that denotes that it has lost its statistical significance.
Coefficients:
Term: Constant
Co-Efficient: 300.165
SE Co-Efficient: 63.10
T-Value:5.666
P-Value:0.000
Term: Velocity
Co-Efficient: 2.120
SE Co-Efficient: 1.1940
T-Value:1.6453
P-Value:0.094
Term: Mass
Co-Efficient: 5.298
SE Co-Efficient: 0.9592
T-Value:5.4323
P-Value:0.000
Term: Energy
Co-Efficient: -23.999
SE Co-Efficient: 1.7986
T-Value:-11.9898
P-Value:0.000
Usually, the coefficient p-values are used to determine which terms are to be retained in the regression model. In the sample above, Velocity could be eliminated.
On the other hand, Regression coefficients characterize the change in mean in the response variable for one unit of change in the predictor variable while having other predictors in the sample constant. The isolation of the role of one variable from the other variables is based on the regression provided in the model.
If the coefficients are seen as slopes, they make better sense, them being called slope coefficients. A sample model is given below for illustration:
Coefficients:
Term: Constant
Co-Efficient: -111.982
SE Co-Efficient: 17.4200
T-Value:-6.5555
P-Value:0.000
Term: Height
Co-Efficient: 106.555
SE Co-Efficient: 11.5500
T-Value:9.23111
P-Value:0.000
The equation displays that the coefficient for height in meters is 106.5 kilograms. The coefficient displays that for every added meter in height you can expect weight to surge by an average of 106.5 kilograms.
Significance of Regression Coefficients for curvilinear relationships and interaction terms are also subject to interpretation to arrive at solid inferences as far as Regression Analysis in SPSS statistics is concerned.
Height is a linear effect in the sample model provided above while the slope is constant. But if your sample requires polynomial or interaction terms, it cannot be intuitive interpretation. In general, polynomial terms structure curvature while interaction terms show how the predictor values are interrelated.
A significant polynomial term makes interpretation less intuitive as the effect of changes made in the predictor depends on the value of that predictor. The same way, a significant interaction term denotes that the effect of the predictor changes with the value of any other predictor too. While interpreting regression analysis, the main effect of the linear term is not solely enough. Fitted line plots are necessary to detect statistical significance of correlation coefficients and p-values. They should be coupled with a deeper knowledge of statistical regression analysis in detail when it is multiple regression that is dealt with, also taking into account residual plots generated.
Contact Us:
UK NO: +44-1143520021
India No: +91-8754446690
US NO: +1-972-502-9262
Email: info@statswork.com
Website: httpwww.statswork.com/
Landline: +91-44-42124284
While interpreting the p-values in linear regression analysis in statistics, the p-value of each term decides the coefficient which if zero becomes a null hypothesis. A low p-value of less than .05 allows you to reject the null hypothesis. This could mean that if a predictor has a low p-value, it could be an effective addition to the model as the changes in the value of the predictor are directly proportional to the changes in the response variable.
On the contrary, a p-value that is larger does not affect the model as in that case, the changes in the value of the predictor and the changes in the response variable are not directly linked.
If you are to take an output specimen like given below, it is seen how the predictor variables of Mass and Energy are important because both their p-values are 0.000. Nevertheless, the p-value for Velocity is greater than the maximum common alpha level of 0.05 that denotes that it has lost its statistical significance.
Coefficients:
Term: Constant
Co-Efficient: 300.165
SE Co-Efficient: 63.10
T-Value:5.666
P-Value:0.000
Term: Velocity
Co-Efficient: 2.120
SE Co-Efficient: 1.1940
T-Value:1.6453
P-Value:0.094
Term: Mass
Co-Efficient: 5.298
SE Co-Efficient: 0.9592
T-Value:5.4323
P-Value:0.000
Term: Energy
Co-Efficient: -23.999
SE Co-Efficient: 1.7986
T-Value:-11.9898
P-Value:0.000
Usually, the coefficient p-values are used to determine which terms are to be retained in the regression model. In the sample above, Velocity could be eliminated.
On the other hand, Regression coefficients characterize the change in mean in the response variable for one unit of change in the predictor variable while having other predictors in the sample constant. The isolation of the role of one variable from the other variables is based on the regression provided in the model.
If the coefficients are seen as slopes, they make better sense, them being called slope coefficients. A sample model is given below for illustration:
Coefficients:
Term: Constant
Co-Efficient: -111.982
SE Co-Efficient: 17.4200
T-Value:-6.5555
P-Value:0.000
Term: Height
Co-Efficient: 106.555
SE Co-Efficient: 11.5500
T-Value:9.23111
P-Value:0.000
The equation displays that the coefficient for height in meters is 106.5 kilograms. The coefficient displays that for every added meter in height you can expect weight to surge by an average of 106.5 kilograms.
Significance of Regression Coefficients for curvilinear relationships and interaction terms are also subject to interpretation to arrive at solid inferences as far as Regression Analysis in SPSS statistics is concerned.
Height is a linear effect in the sample model provided above while the slope is constant. But if your sample requires polynomial or interaction terms, it cannot be intuitive interpretation. In general, polynomial terms structure curvature while interaction terms show how the predictor values are interrelated.
A significant polynomial term makes interpretation less intuitive as the effect of changes made in the predictor depends on the value of that predictor. The same way, a significant interaction term denotes that the effect of the predictor changes with the value of any other predictor too. While interpreting regression analysis, the main effect of the linear term is not solely enough. Fitted line plots are necessary to detect statistical significance of correlation coefficients and p-values. They should be coupled with a deeper knowledge of statistical regression analysis in detail when it is multiple regression that is dealt with, also taking into account residual plots generated.
Contact Us:
UK NO: +44-1143520021
India No: +91-8754446690
US NO: +1-972-502-9262
Email: info@statswork.com
Website: httpwww.statswork.com/
Landline: +91-44-42124284